Introduction: Into the Black Box of Google Translate
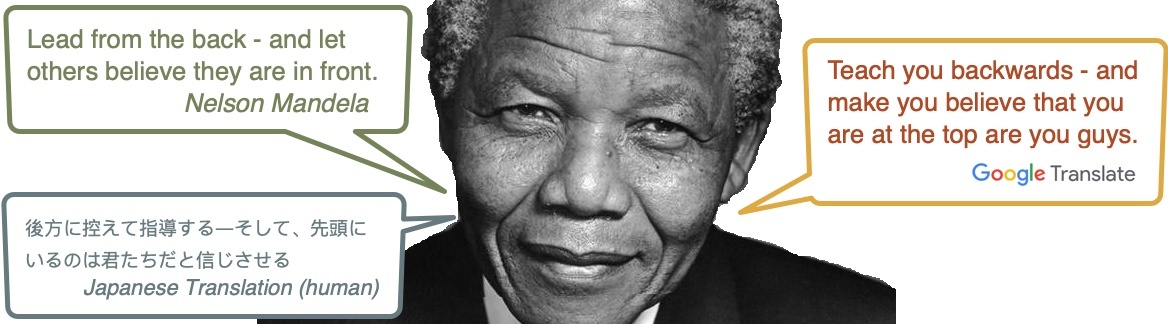
Picture 1: Google Translate translation. The top left is an original English quote from Nelson Mandela. Bottom left is a human translation of Mandela’s quote to Japanese. On the right is Google Translate’s translation of native Japanese back to English. (誰もが知るべきネルソン・マンデラの17の知恵, translated by Koichi Higuchi.) Human translations of this quotation to many other languages, and then by Google back to English, including Mandela’s mother tongue Xhosa, are collected at http://kamu.si/mandela-lead
Does Google Translate translate? You might put your faith in it. You might laugh at it. I studied it, for all its languages. This is the first comprehensive examination ever conducted of the world’s most-used translation tool.
The research before you was not intended as an exposé of “fake data” in a multi-billion dollar industry. I set out with a difficult but narrow question: what is the quality of the results produced by Google Translate (GT) among the 108 languages it offers? Answering the first-order question about GT, though, led inexorably to an examination of the integrity of the claims underlying their version of machine translation (MT).1 I found that, with certain types of formal texts for a very few language pairs, GT often produces remarkably good results. In the vast majority of situations for which they make claims to deliver something they label translation, however, the results go beyond imperfect. They are algorithmically designed to produce output data without any basis in linguistic science, often divorced from any actual form of human expression.
Nobody has empirically analyzed the internal workings of GT before because it is too complicated to figure out what is going on inside, with so many languages that no individual or small team could see into. As a result, the media and the public have long taken Google’s bold claims at face value, the way everybody believed Volkswagen’s “Clean Diesel” deception because nobody had developed the means to test their emissions outside of constrained conditions, until a research team took some VWs on the road and uncovered a massive toxic fraud . An arduous investigation of Enron’s opaque books unearthed a similar scandal, finding that the erratic delivery of electricity to customers in California overlay the defrauding of global investors of billions of dollars . While the economic stakes are not as high – VW paid over $25 billion in fines, and Enron went bankrupt – a journey along the threads of my original research question revealed a business enterprise that deliberately fakes the data to defraud its users by the billions of words every day.
Moreover, as I dug deeper, the research began to call into question many of the major tenets of the entire branch of computer science devoted to translation. Academic journals and the popular media are both full of ballyhoo about the miracles offered by Artificial Intelligence (AI) and the hot trends that will get us there: neural networks, neural machine translation (NMT), machine learning, deep learning, zero-shot translation. Many people take it for granted that we can someday achieve perfect universal translation using these techniques, if we haven’t already. My investigations found quite the opposite: MT on its current trajectory cannot possibly reach the promised land, because it has neither adequate data nor adequate techniques for converting the data it has across languages. This book debunks myths about AI (Myth 1), NMT (Myth 2), zero-shot (Myth 3), and computer omniscience (Myth 4).
Additionally, an entire chapter looks at the relationship between words, numbers, and translation, to explain the difficulties that will always prevent GT and its kin from achieving the results they promise. Please do not be daunted – the discussion is not as heavy as the word “mathematics” in the chapter title might imply. You will learn a lot about MT if you go there, but the hit counter shows people are scared that 👻👺☠👹💀 lurk there.
My line of work is language, or, better said, languages. All of them. A progression of events stemming from anthropological efforts to understand the persistence of poverty in Africa put me on an unlikely journey , the modest goal being the creation of a complete matrix of human expression across time and space. For over a quarter century now, I have been working toward this goal with language specialists, computer scientists, and citizen linguists,2 developing systems to collect and process all that is knowable about the words people speak. Along the way, I have run into one consistent question: “Doesn’t Google already do that?” The answer is emphatically no. They don’t, and they can’t. However, “no” is an inadequate answer, so I set out to discover, through empirical research, what it is that Google actually does. What follows are the results of that project.
This is the age of “fake news” and “alternative facts”. The morning starts with presidential prevarication on Twitter, or the White House lawyer arguing “truth isn’t truth” . Facebook sells your personal data while telling you it protects your privacy . Top-ranked search results promulgate medical misinformation, with sometimes fatal consequences . Much of the Internet is populated with fake businesses, fake people, and fake content . You yourself are complicit in the culture of lying each time you agree that you have read the terms of service for the apps and websites you use (see Picture 2).
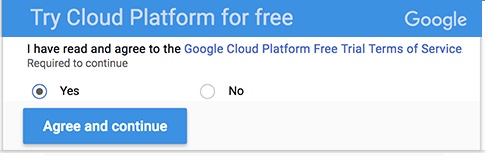
Picture 2: A checkbox from Google mandating an attestation that, through click history, both parties know is false. All 5 FAAMGs (Facebook, Amazon, Apple, Microsoft, and Google) have services that force users to click next to the words “I have read … the terms” in order to continue.
Google claims that it produces accurate translations among 108 languages. Is Google Translate fake data? It’s complicated. In all languages, some data is real and some is invented. In most languages, most data is3 fictitious.
The takeaway: For 36 languages vis-à-vis English, GT is better than a coin toss, producing text that conveys the main idea more than 50% of the time. For languages at the very top of TYB tests, if five conditions are met (listed in the conclusions – here’s a wormhole), you will often receive conversions that transmit the meaning, with good vocabulary and grammar, though there is always serious risk of a serious error. For 71 languages – over two thirds of the languages they claim to cover – GT fails to produce a minimally comprehensible result 50% of the time or greater. For translation between languages where neither is English, the gist of the original is transmitted in approximately 1% of pairs. As to “universal translation” , GT does not touch the remaining 6900 of the world’s roughly 7000 languages: emphatically no. Full data is available at http://kamu.si/gt-scores.
To make use of the ongoing efforts the author directs to build precision dictionary and translation tools among myriad languages, and to play games that help grow the data for your language, please visit the KamusiGOLD (Global Online Living Dictionary) website and download the free and ad-free KamusiHere! mobile app for ios (http://kamu.si/ios-here) and Android (http://kamu.si/android-here). How much did you learn from Teach You Backwards? Your appreciation is appreciated!:
)
Readers’ Note: Repeated acronyms and technical terms can be accessed from the “About” menu at the top of every page. Please also take the informal and colorful expressions used throughout this text as intentional suggestions for your own tests of GT’s translations of everyday English into your chosen language. To notify TYB of a spelling, grammatical, typographical or factual error, or something else that needs fixing, or a reference that should be added, please select the problematic text and press Ctrl+Enter to open a correction submission box.
Overview4
I have led an in-depth independent study of Google Translate (GT) from both quantitative and qualitative perspectives. Please read my notes about the genesis of this research and the style in which it is presented; this web-book is a hybrid between scientific and investigative reporting that strays outside academic conventions in the effort to tell the story of GT both to experts and to its millions of users outside the ivory tower. The primary research across all 108 GT languages is described in detail in the Empirical Evaluation, as an objective scientific essay that transparently details the research, and releases the results as open data. The Empirical Evaluation is intentionally separated from the more opinionated parts of this book, which interpret the results, explain why you should care, present a path toward more honest and effective MT, and suggest do’s and don’ts for your relationship with GT. This is the first comprehensive analysis of how GT performs in each language they offer in their current configuration.5 Within my qualitative analysis, I further report the results of a four year study across 44 languages of GT’s public contribution system that they say is used to improve their services. Additional questions are answered herein with research among smaller sets of languages.
Journalistic parts of this web-book look at the facts and the fantasies surrounding artificial intelligence (AI) and MT, showing from an expert perspective what GT is capable of versus what it does not and can never achieve. I determine that Google Translate is a useful tool among certain languages when used with caution, but I also show scientifically when, how, and why its truth often isn’t truth. After the qualitative conclusions, I offer detailed recommendations about the contexts in which you should use it and the situations where it cannot be used, including hazard warnings to never use GT for blind translations in any situation that matters, never use GT as a dictionary for individual words, never use GT to translate poetry or jokes, and never use it to translate among non-English pairs. I further advocate that GT should be required to print a disclaimer on all its output stating that the service must not be used in medical situations; where garbled translations could lead to serious harmful consequences, GT’s initially high failure rate for most languages is exacerbated by its lack of training in the health domain, and qualified human translators are essential.
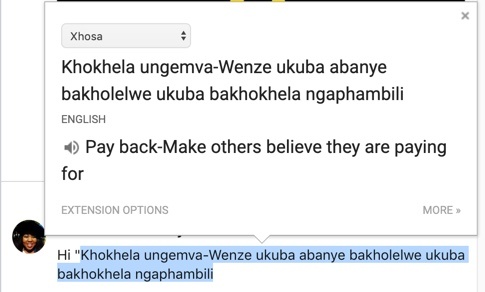
Picture 2.1: “Lead from the back – and let others believe they are in front”, translated to Xhosa, Nelson Mandela’s mother tongue, by Xhosa scholar dozens of GT languages.
, then translated back to English by GT. This translation experiment has been performed acrossSometimes GT offers translations that are indistinguishable from those a person would produce. Sometimes, the service offers clunky, fingernails-on-a-blackboard phrasing that nevertheless translates meaning across languages. Quite often, though, GT delivers words that can in no way be considered meaningful translations, such as transforming “lead from the back” into “teach you backwards” from Japanese (see Picture 1) or “pay back” from Mandela’s Xhosa mother tongue (Picture 2.1). The book before you opens the lid of GT’s sealed black box, to examine what the system actually does in relation to how it is portrayed and understood.
GT, embedded in Google’s market-leading search results and Android operating system, as well as numerous third-party services, positions it as the world’s go-to service for automatic MT. Ubiquity does not indicate sagacity, however. The widespread belief that Google has solved MT could hardly be farther from the truth. From English to the 102 other languages they attempt – their bread and butter – Google’s reliability is good in a few, marginal in many, and non-existent in many others, in the tests I conducted. Translations among all but a handful of non-English languages in the GT set are persistently unreliable, being based on a conversion through English that compounds the error rate on both sides. Nonetheless, Google makes both explicit and implicit claims that GT can consistently translate as well as people. Hugo Barra, a vice-president of Android, Google’s software for mobile devices, says Google’s system is “near-perfect for certain language pairs”, such as between English and Portuguese . On their tenth anniversary, GT stated on their blog:
Ten years ago, we launched Google Translate. Our goal was to break language barriers and to make the world more accessible. Since then we’ve grown from supporting two languages to 103, and from hundreds of users to hundreds of millions… Together we translate more than 100 billion words a day… You can have a conversation no matter what language you speak.
Importantly, these claims underlie not only the free public access mode with which most people are familiar, but also Translation API, a product that Google sells for real money. At https://cloud.google.com/translate, they state, “Translation API supports more than one hundred different languages, from Afrikaans to Zulu. Used in combination, this enables translation between thousands of language pairs.” Picture 3 shows pricing information for bulk users of GT services. Google specifically tells paying customers that they produce valid translations among thousands of language pairs, entering into contracts with their customers in which the output GT delivers in exchange for money is represented as “translation”. I doubt that anybody in South Africa has ever considered paying GT to translate from Afrikaans to Zulu (both official national languages) after one glimpse at the results, but anyone who has paid for translation among such pairs based on Google’s explicit claims has fallen prey to a swindle, buying a product that my research shows is fraudulent by design.
In certain cases that will be discussed in this web-book, GT produces fully comprehensible results. Google’s best performances between English and its top tier languages for texts in some formal domains, however, leave many with the erroneous impression that similar results are produced consistently for all text genres in those languages, for all non-investment languages versus English throughout its list, and that such results are also achieved among language pairs when English is neither the source nor the target.6 These assumptions are based on several tropes and mind tricks that lead to outsized confidence in the words GT offers as translations.
Tropes and Mind Tricks that make you believe in make believe7
- Elite language bias.8 The studies, demonstrations, and personal experiences that most active users of GT are aware of involve fewer than a dozen market languages on which the service has focused considerable attention: the FIGS, Portuguese, Russian, Chinese, and a few other European languages that, not coincidentally, score among the highest in my tests. Google’s public demonstrations showcase the languages where they feel the most confident. People who have only witnessed GT in elite languages often assume that the same level of performance occurs throughout. Until this study, no research I know of has examined GT’s performance on most of the dozens of languages that the audiences in their lucrative markets would have no occasion to encounter.
2. English speakers see only English.9 GT consumers typically consider it a service where English is either the source or the target. That is, “translation” in GT equals translation versus English. Not coincidentally, English is the lopsided focus of natural language processing (NLP) research, regarding both its internal dynamics and translation to other languages. English is therefore the showroom model, with much more window dressing than the languages on the outskirts. Much as you could get iced green tea at Starbucks but you generally go there for coffee, you know you could try Samoan to Somali in GT but you probably won’t.
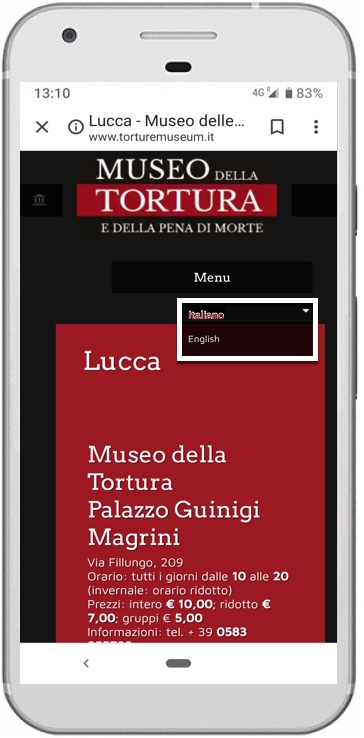
Picture 3.1: Website for the Museum of Torture in Lucca, Italy, where translation is provided only in English. Lucca is jam-packed with tourists from around the world, especially from Asia, who cannot read either English or Italian. (Language selection box slightly photoshopped to improve visibility.)
Demographically speaking, English prevalence worldwide is very much like left-handedness, homosexuality, and vegetarianism – it occurs everywhere, and in similar numbers. To the extent that GT performs best only between English and a handful of other languages, its grand success is a service for gay vegetarian lefties. Casting no aspersions against that select group, it is surely only a small portion of global translation needs. English speakers tend to vastly over-estimate the number of people worldwide who speak their language, for several reasons:
-
- If you go to a hotel or upscale restaurant in any city with an international airport, the staff will speak English – it is a job qualification, the way knowledge of drug interactions is a requirement to work in a pharmacy.10 Some people everywhere learn English, and many of those people will seek jobs where their English is useful, and they will be hired favorably by employers who serve people from abroad. If you find yourself needing to talk to a police officer or a hairdresser, you will be much less likely to find competent English, because learning the language is not a necessary skill for most people training for those professions.
- A few countries, especially in Northern Europe, make a point of teaching English to their youth. People who’s major travel experience is the Netherlands might barely even perceive that Dutch is the national language. On a trip to Norway, I could always find a friendly young person to help me, for example, read the ingredients on a bottle of pasta sauce to figure out if it was vegetarian. Even in Norway, though, many English interactions were strictly limited – a young agent at the train station digging deep for “follow her” to point me toward the transport I needed, or, when three women in a fjord swam past me on a dock where I had a good view of hazards in the water, one was able to put together, “Are there jellyfish?” India has tens of millions of English speakers, but my experience interviewing students for IT positions shows that even the level of brilliant techies is often far less than brilliant. A colleague at a major international organization once assured me that “everyone in India speaks English”; when I visited her home in Delhi, I found that “everyone” did indeed, but not their driver, not their gardener, and not their cook.
- People who travel are also more likely than most in their country to speak English, either because they like traveling and learned English to make their movements easier, or because they belong to a class where both English and international travel are part and parcel.
- People with international professions, including academics, usually learn English as the common language of communication. This is a small minority of people in most countries, but a majority of the people a professional is likely to associate with. It is an absolute requirement for pilots and air traffic controllers, since disasters would result without an agreed common language, so your pilot will almost always make some in-flight announcements in English.
- You will also think that tons of English is spoken because your ears naturally perk up when you hear sounds you recognize, in a way you would not notice someone behind you speaking Polish or Lao or Hausa, unless you are Polish, Laotian, or Nigerian. My ears will pick out the dulcet tones of an American from 40 feet away. Recently, though, I was at a Franco-Italian wedding in Switzerland where the woman seated to my left could not identify what language I was speaking with my family, who were the only English speakers on the guest list.
- English is a language people aspire to. Parents in many parts of Africa are eager for their children to learn it, and many European youth choose it for their language requirements in high school. From Korea to Ecuador, many people have learned enough English to ask you “How are you?”, but not nearly enough to really tell you how they are. Nevertheless, minor interactions with people who can eke out some pleasantries provides confirmation bias that the whole world is speaking the language. Having meandered widely outside of English mother-tongue environments for much of my life, I have witnessed frequently that there is much less of the language than meets the ear.
- English makes many cameo appearances in places where it is not actually understood. People worldwide wear T-shirts that bear English messages, some of which make sense. A dinosaur-skeleton keychain from Japan that I used for years had the message “Joyful Stegosaurus Happy Bone”, which looks like English. Advertisers throughout Europe often use English or English-esque taglines to give an international or youthful flare. Public buses in Thessaloniki announce all their stops: “επόμενη στάση [stop name], next stop [stop name]”, as though (a) no foreigners would cotton on that “epómeni stási” is Greek for “next stop” by the third time they heard it, and (b) all foreigners would understand “next stop”. Products everywhere feature switches labeled “on/off”, but mastering the on/off switch is a far cry from mastering English – and even this text is being replaced by a universal icon. In places far and wide, English words often float through the air like soap bubbles shimmering in the breeze.
- English is like the US dollar. It is the most common global medium of exchange, but that does not mean that most people hold, or have ever even seen, dollars. Elites from every country use dollars for international transactions, but they use the local currency and the local language for their local activities. Americans in particular assume that the world runs on the greenback. I once stood in line behind an American who had just arrived at London Heathrow airport, and, when he demanded a train ticket to Buckingham Palace, was outraged that the clerk would not accept his money. For sure, when dealing with funds for Kamusi, the US dollar is essential to pay for IT and linguistic work in places like India and Kenya, or sometimes even Switzerland. However, when I go grocery shopping in Switzerland, or anywhere else on the European continent, my dollars are always useless, and my English is only marginally more likely to oil the transaction.
Until this study, third-language translations have gone almost entirely unexamined, with viable translations of formal texts from a few elite languages to English serving as a synecdoche for all other cases. Although anybody reading this article obviously has a sophisticated knowledge of the language, only 15% of people speak English somewhere on the spectrum from knowing to get off the bus when they hear “next stop”, to conducting research in nuclear physics. For 85% of the world, English rests as far from their daily experience as deep-sea fishing probably does from yours, though access to dependable translation of the large chunk of global knowledge that is housed in English would benefit speakers of any other language.
A sense of English competence around the world can be gleaned from the EF Proficiency Index, which ranks countries by English skills on the basis of online tests of people interested in online English classes (not a representative measure!). I’ve been to 34 of the top 50 countries on the list, and can attest that moving around the Netherlands (#1) in English is simplicity itself, but getting a haircut in urban Portugal (#11) involves a lot of pointing and hand waving, and no English words will help find out what’s going on with a diverted train in southern Bulgaria (#24).
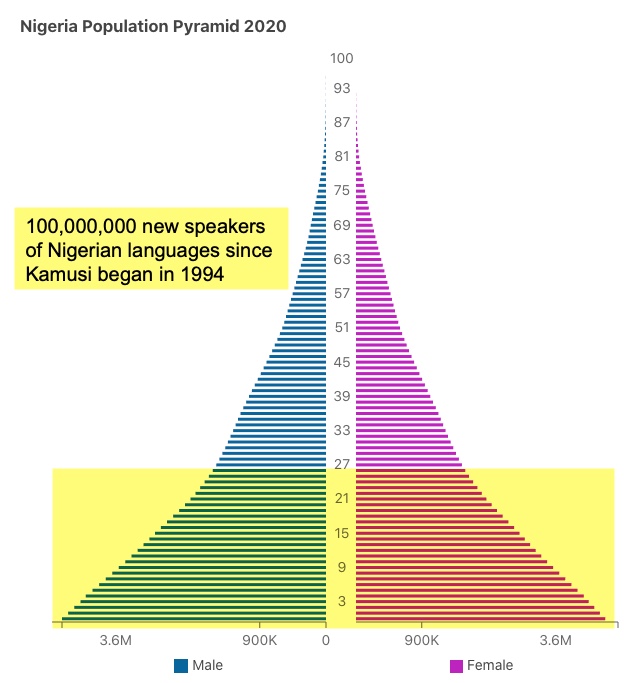
Picture 3.2: The population of Africa has roughly doubled in the past quarter century. This graph from World Population Review shows the number of people born into African language communities in Nigeria during that time.
Meanwhile, the population of people born into many non-English-speaking communities as much as doubles every twenty-five years. Nigeria, for example, had roughly 108,000,000 people in 1995, and 207,000,000 million in 2020, as shown in Picture 3.2. Although the “official” language of Nigeria is English, those youth are mostly speaking Yoruba or Igbo or Hausa or others of more than 500 languages. The percentage of the population age 15 and above who can, with understanding, read and write a short, simple statement on their everyday life in any language, a.k.a. the literacy rate, was estimated at 62% in 2018. What proportion of those people have basic literacy in English is unreported, but only about 45% of Nigerians attend even a year of secondary school, which is where students would be expected to aim toward proficiency. Many African countries have similar demographics, but only some have English as their colonial souvenir language. Most countries with similar population growth in Asia and the Americas have almost no official relationship to English. We can reasonably posit, then, that many hundreds of languages are growing rapidly in terms of raw numbers of speakers, while English could actually be losing ground in terms of the percentage of people globally who speak it.
English is thus an important but fairly small part of the potential global demand for translation, with geographically proximate pairs such as Chinese-Japanese, Hindi-Bengali, Yoruba-Swahili, and Quechua-Aymara often the mixes that matter to the roughly 7 billion people who cannot read this paragraph. Using round numbers, the current global population is 7.7 billion people. Although reliable census data does not exist, the rough guess from knowledgable people hovers at around 5% as native English speakers, not all of whom are literate. Roughly 10% of others are guessed to have some knowledge of the language. If half of second language learners have functional literacy, we can posit around 700 million readers of English, and 7 billion for whom English is just background noise.
3. In Google We Trust.11 People have implicit faith that, in general, Google delivers what it says it will, be that websites, map locations, images, or translations. All users will share Hofstadter’s experience: “if I copy and paste a page of text in Language A into Google Translate, only moments will elapse before I get back a page filled with words in Language B” . The fact is, though, that you never blindly accept the first photo proposed by Google Images. You accept that the results are all indeed pictures, but you are able to use your own judgement to assess which of hundreds of images best meets your needs. If you were to search for a picture of “wild turkey”, the bird in Google’s first result, Picture 4, might meet your exact desires, or perhaps you prefer a different image from their bird candidates, or maybe the bottle of bourbon that appears seventh will better quench your thirst. Your confidence in the service derives from their ability to unearth a host of images for you to choose from, even if sometimes they miss completely. If you are actually looking for a picture of Turkish wilderness that would resemble the results for “wild bulgaria”, their decision that you want birds or bourbon is a fail, and you hone your search with a term such as “mountain” or “forest”. People blindly accept text from GT, however, because they do not have the linguistic skills to evaluate the proposed translations. It would be unimaginable to accept the first suitor chosen by a dating website – services like Tinder revolve around users swiping left to reject matches the algorithm proposes – but that is the confidence that many users accord the basket of words delivered by GT.
Research by Resende and Way demonstrates that students trust Google so much that they often use it as a tool for language learning: “Participants trusted the GT output enough to change their linguistic behaviour in order to mirror the system’s choices.” In their carefully controlled trials, they found that students adopted a grammatical pattern that Google produced consistently from Portuguese to English, which was an improvement versus replacing Portuguese words with English words while maintaining the Portuguese grammatical pattern. The authors go on, though to “wonder whether MT pitfalls can be learnt and generalized by users when speaking or writing in English.” TYB shows that GT gets it wrong in the proportion of cases demonstrated in the empirical findings, yet Resende and Way show that students often trust the service to teach them the proper way to speak a foreign language. What could possibly go wrong?
4. The willing suspension of disbelief.12 Although people see faulty translations in GT time and again (the “Translation Fails” channel by Malinda Kathleen Reese on YouTube contains numerous examples), they credulously assume the next translation will somehow work. This is the psychology of desire over evidence. For low-scoring languages, many people seem to take pride in the inclusion of their language within the service, though their experience shows that it is of no value in their own translation needs. For example, though he scored his own language a zero in my tests, a respondent still wrote, “I also would like to thank Google for including my language (Tajiki) into this 102 languages on their online service”. An evaluator for Kinyarwanda who assessed the results as “misleading”, “inadequate”, and “strange”, continued, “Despite all these, the tool is amazing when I consider being without it. It psychologically gives you an impression of hope of having an improved version as time goes on”. Similarly, though Hawaiian failed 75% of the time in my tests, Aiu quotes a Hawaiian student named Hōʻeamaikalanikawahine as saying, “To see our language thriving and being recognized by Google makes me proud. I imagine that our kūpuna who got beaten for speaking Hawaiian in school, but continued to speak Hawaiian in order to make sure the language was not lost, are also standing proud right now. Their bravery and perseverance resulted in the revival of such a beautiful language. I’m hoping that as more people see, hear and use our ʻŌlelo, they become familiar with the idea that Hawai’i is more than just a tourist attraction, but the home of a thriving and healthy language.” This might be called the “Fleetwood Mac effect”: “I’ll settle for one day to believe in you… Tell me sweet little lies”.
5. “It isn’t perfect, but…”13 I have heard these exact words repeatedly, and for people who use GT for informal translations in languages at the very top of the heap, it might be a worthy sentiment. For example, a Sudanese woman who works in the planning department at UNICEF in Geneva said, “It isn’t perfect, but…” in regard to her use of the service to convert to English for things she cannot understand in French. However, she chuckled derisively when asked whether she uses it for her native Arabic. A British simultaneous translator from English to Spanish for the International Olympic Committee also used the phrase, explaining that he uses GT when he gets the text of a speech at the last minute so he can quickly see if there is tricky vocabulary coming his way. The first Google hit for “google translate accuracy”, the website of a translation agency, concludes GT is good for casual use but urges human translation when the result is important: “It gets the general message across, but is still far from perfect.” 14 Phrased a bit differently, “Google Translate’s pretty good but it’s not always accurate”.15 I could go on.
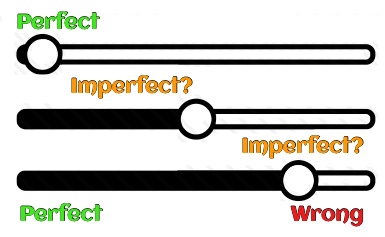
Picture 4.1: The imprecision of imperfection. When something “isn’t perfect, but…”, where does it fall on the scale between perfect and perfectly wrong?
Framing the results in terms of “perfect” introduces a matter of psychology. I’ll do some mind reading by predicting your answer to the question “what is the opposite of ‘perfect’?”. You said “imperfect”, right? The term blocks you from considering any other antonym. But what is “imperfect”? If my daughter were to bring home a German exam where she lost one point for an errant umlaüt, that would be imperfect. If she were to bring home the same exam with only one word right, that too would be imperfect. When evaluating translations, “imperfect” is somewhere between 1% and 99%, and the opposite of “perfect” is “wrong” (see Picture 4.1).
Many people do not expect perfect translations from GT, and feel that getting some of the words is better than nothing. We often get satisfaction from the effort, not the result, such as the pleasure a parent feels at a child’s music recital or sports event despite the distance from reaching professional standards, or the conviction that their child is a genius the half the time that they correctly say something is left versus right. This is church coffee : free and well-intentioned, which people drink even if it tastes like it was brewed in the mop bucket. Although a Wired review of the GT “Pixel Buds” product a year after its release evaluated it as “a bit of a con” (Leprince-Ringuet 2018), this piece of journalism, where failure is techsplained as a raging success, accompanied its release:
The coolest part of [Google’s] Pixel Buds is the ability to use them as a universal translator. It’s like something out of “Star Trek” — at its November launch, you’ll be able to use the Pixel Buds to have a conversation across 40 languages. I had the chance to try this feature out. And it works! Mostly. Now, the magic happens. In my demo, I tried out my mediocre Spanish on a Google spokesperson wearing Pixel Buds, so I’ll use that as my example. He spoke English into the Pixel Buds, asking “hi, how are you?” The Pixel 2 phone spoke, out loud, the equivalent Spanish phrase: “¿hola, como estas?” This text was also displayed on the screen, which is good, because the demo area was noisy. That noisy room also led to the demo’s biggest glitch: When I went to answer in Spanish — “muy bien, y tu?” — the Pixel 2’s microphone didn’t pick me up clearly. In theory, my conversation partner should have heard “very well, and you?” Instead, all the app heard, and translated, was “William?” Bummer. I’m willing to cut Google some slack, here — the room was cacophonous with the sounds of my fellow tech reporters playing around with all of Google’s new gadgets. In my own experiences with Google Translate, it’s pretty solid at recognizing language, so I trust that it would work as well here. Still, be aware that it might not work in a noisy bar… It’s another sign of how Google is turning its considerable edge in artificial intelligence into futuristic, but very real products that make a difference today.
6. GT learns constantly from its users.16 The impression that GT has a steady program to improve based on input from users. GT says they are doing this through the promulgation of the “translate community”. I conducted a four year experiment of their crowd contribution process among 44 languages, with more than 200 volunteers, and find it to be largely ineffective and inherently flawed, contravening scientific requirements for linguistics and computer science. There is some learning going on – for example, about a year after I submitted an error report, the wrong translation of “Tutaonana” from Swahili to English shown in Picture 37 was eventually corrected to “See you later” if entered by itself in uppercase, or “we will see each other” if typed in lowercase (both valid though my suggestion was the all-purpose “Farewell”, so these suggestions came from elsewhere), but mutilated when put into the formula that closes millions of phone conversations every day (“Haya, tutaonana” is rendered as “Well, we’ll see”, instead of “Ok, goodbye”). The belief that GT learns from its users is the fifth myth investigated in the chapter on qualitative analysis .
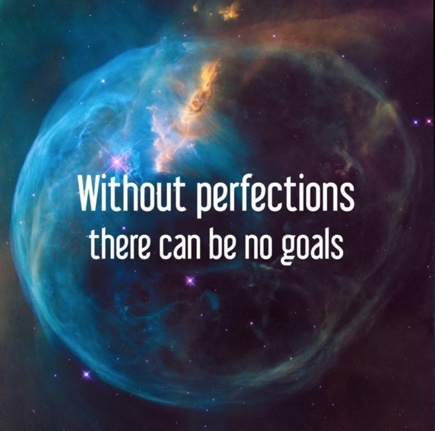
Picture 5: Robot-generated text to which humans might ascribe meaning. (http://inspirobot.me)
7. Don’t mind the gaps.17 People are naturally inclined to skip over gaps in meaning. If a cluster of words seem like they should make sense, people will twist their minds to give them sense. The effect is well illustrated at InspiroBot, which generates fake inspirational quotes such as “Be in support of the wind”, and “Expect eternity. Not your inner child”, and the pseudo-profundity in Picture 5. Peder Jorgensen, co-creator of the site, ponders, “Our heads, our minds, you try so hard to give them meaning, so they start making sense in a way,” and his radio interviewer adds, “It’s not what the words say. It’s what they say to you” . It is apparent from comments by participants in this study that machine translation from English “works” a lot better for people who already know a substantial amount of the source language. For example, a Polish evaluator who lives in the US was able to apply her stock of American metaphors to the Polish word-by-word conversions of English expressions, and consider them fine translations, whereas a Warsaw-based evaluator did not have the parallel English conceptual framework and therefore had no clue about what motivated the translation. Similarly, evaluators across languages with substantial English and some familiarity with gay culture were better prepared to understand a literal translation of “out of the closet”, despite the non-existence of that metaphor in their own society, than people who had never encountered the English phrase. In the words of Hofstadter , “It’s hard for a human, with a lifetime of experience and understanding and of using words in a meaningful way, to realize how devoid of content all the words thrown onto the screen by Google Translate are. It’s almost irresistible for people to presume that a piece of software that deals so fluently with words must surely know what they mean”.
8. Confirmation bias.18 You might have a hunch about how a phrase should be translated. For example, you might think that “spring tide” has something to do with the seasons. When GT gives you a result that uses a term for the spring season, your hunch seems to be verified. GT is wrong, but you judge that it is right because its proposal matches the guess you would make yourself. Even more significantly, GT often does get all or part of a translation right. We often register the wins in the victory column, but shrug off the misses, with the lasting impression that the overall quality is higher than shown by empirical tests.
9. Gaslighting.19 If you have weak skills in one of the languages in the translation pair, you might accept something from Google that does not look instinctively right. Maybe you question the translation, but then you question yourself for questioning it. Maybe GT knows something you don’t? After all, they’ve analyzed millions of documents and you struggle with a newspaper article. Who are you going to believe, the supercomputers at Google or your lying eyes? This functions in the same manner as your belief in your GPS; surely you have been led down cow paths when your intuition told you to stay on the main road to reach your destination, but, nevertheless, you find yourself turning onto every future cow path your device directs you toward.
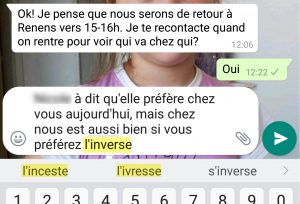
Picture 5.1: When arranging a playdate for my daughter, with the phone set to French, the autocorrect suggestions were incest or drunkenness. NLP for investment-grade languages like French does not generally reach the same level as English. For non-market languages, ivresse is a pretty good descriptor.
10. We are suckers for hype.20 We are repeatedly led to believe that GT and its competitors21 are delivering valid translations because the media tell us so, because Google tells us so (see Picture 6), and because its fellow members of FAAMG tell us so. “Google says its new AI-powered translation tool scores nearly identically to human translators” , say the headlines, and “Google Announces New Universal Translator” . Please read the transcript and watch a presentation where Facebook’s Chief AI Scientist states as fact that we have essentially solved MT among all 7000 human languages, a proposition that I have heard echoed by many other computer scientists who should know better. (The many errors in his claim are dissected as Myth 1 and Myth 2 in the qualitative analysis; click the footnote to read a Facebook translation from Chinese to English that conveys that the writer went toy shopping, but does not indicate Facebook has licked MT.22) “One of our goals for the next five to 10 years is to basically get better than human level at all of the primary human senses: vision, hearing, language, general cognition”, says Facebook CEO Mark Zuckerberg . Similarly, in one podcast, David Spiegelhalter, a deservedly well-respected slayer of myths about risk and statistics at the University of Cambridge said, “There are algorithms that you want to operate completely automatically. The one that when you pick up your camera phone it will identify faces… the machine translation, the vision, they’re brilliant, and they all work, and I don’t want to have to ask them every time, ‘why do you know that’s a face?’” . Prior to this study, you had no tool to evaluate the hype on your own, so you can be forgiven for taking wild exaggerations about GT at face value. Now that you have this study’s empirical results and qualitative analysis at hand, you have the basis for a more realistic understanding of the hype that is spewed about the words GT spawns.
Late-breaking news (24 September, 2019): In my experience, travel for intergovernmental agencies is often finalized at the last minute. As I write, I am waiting for tickets for such a trip, for a meeting in a few days. The last time I flew for this agency, the ticket was confirmed as I sat on the train to the airport. In response to my latest email hoping to prevent a repeat of history, today I received a reply, “Notre agent de voyage émettra tous les billets ce jour même.” I was fine with the first part, but, the most frequent French term for today is “aujourd’hui”. “Ce jour” is a common alternative, but I knew that mixing it with “là” could change the meaning to “that date”, and I had never encountered a construction with “même”, French for “same”. Would my tickets be issued today, with enough time to pre-order vegetarian meals for the 12 hours in the air, or was I being told that I should make my way to the airport check-in desk for a ticket to be issued on the same day? From GT: “Our travel agent will issue all tickets that day” (Bing and DeepL said that same day). This is an important communication, from one of GT’s highest-investment languages to its English heart. “Today” is the 214th most common word in the English corpus. GT is using an NMT model for French that we are assured is nearly flawless, and getting better every day. And yet, I had to wait until I picked up my daughter after school to get the native-speaker interpretation, “today”, reassuring me that maybe tomorrow I’ll have confirmed seats. As to “late-breaking news”, GT gives a few variations from English to French, depending on how you capitalize, hyphenate, and punctuate the phrase, ranging from fine to freaky, but delivers the equivalent of “news about being late” for other languages. One major language pair (English-French), two common terms that needed translation in the normal course of a day (today and late-breaking news), two cases where the meaning is important (getting an intercontinental flight, and publishing for a global audience that includes many non-native readers), two instances where the result is dubious. Words have been rendered, but you should not trust that translation has occurred.
Google Translate’s Android app has one oft-touted feature that is cool to use, but is not actually related to translation. You can point your camera at any written text, such as a sign or a menu, and the app will overwrite the text with a translation, simulating the original design characteristics (font, color, size, angle, etc). The underlying technology combines image processing, optical character recognition, and language identification (which Google does very well, except for some difficulty with, e.g., texts from the Wikipedias for closely related languages like Bosnian and Croatian). Once the image has been parsed as text, it is sent to the standard GT translation engine, then returned to the pixel processor to overlay the original text with the translated words in an emulation of the original format.
It is important to note that Instant Camera results are at best only as good as the translations that would result from typing the same text into the input box, rather than taking a photo. Results are often degraded when parts of the text are not properly recognized by the imaging software, as happened in some of my informal tests with Google’s flagship Pixel 3 phone. Discussing the imaging technology in the same breath as discussing the translation technology is reminiscent of answering the question “How was your flight?” by saying, “I had a Coca-Cola in a plastic cup from the beverage cart. It was sweet and tangy and really quenched my thirst” – completely tangential to the question at hand. Picture 5.1.1 shows a German menu from a restaurant in Heidelberg, and Picture 5.1.2 shows the Instant Camera translation to English. You might not know the Japanese on a food package attempted by @droidy on Twitter, but you do know that Instant Camera is winging it with “The god is Pakkane” or “finger is in a paging cage” as she was shown. A Kenyan journalist reacts to the introduction of the technology for her language with due perspective: “a quick trial of the new feature doesn’t seem to produce the touted accuracy in certain instances, but we all know how poor Swahili translations can be from our experiences with Twitter and Netflix.”
I suggest a trial that you can do on your own. I did it with a box of herbal tea from a local supermarket, where the ingredients and instructions were printed in parallel in German, French, and Italian – you can probably find something similar in your own pantry, or you can use a multilingual instruction manual you have saved for some gadget. For my test, I used the GT app to take a photo of the Italian portion of the tea box and translate it into German (both high scoring languages in TYB tests). There was very little room for ambiguity. Nevertheless, the German results were unusable. Many of the words were right (including “Maltodextrin” for “maltodestrina” – nice get!), but the overall output was a hodgepodge of words, fragments, and isolated letters. The sentence meaning “Store in a cool dry place” came into German at a Tarzan level, with a word choice indicating one should put the tea in the basement and forget about it. This was a good exercise because it displayed GT results in one hand on the phone, and professional results in the other hand on the box. The teabox experiment had too much text to lend itself to photography, though, so I followed my own advice and tried a more photogenic instruction manual for walkie-talkies that had been professionally translated by Motorola. Picture 5.1.3 shows the results from Hungarian to English. You can judge whether you understand the translation from Hungarian in the center to give you the same information as the original English on the left.
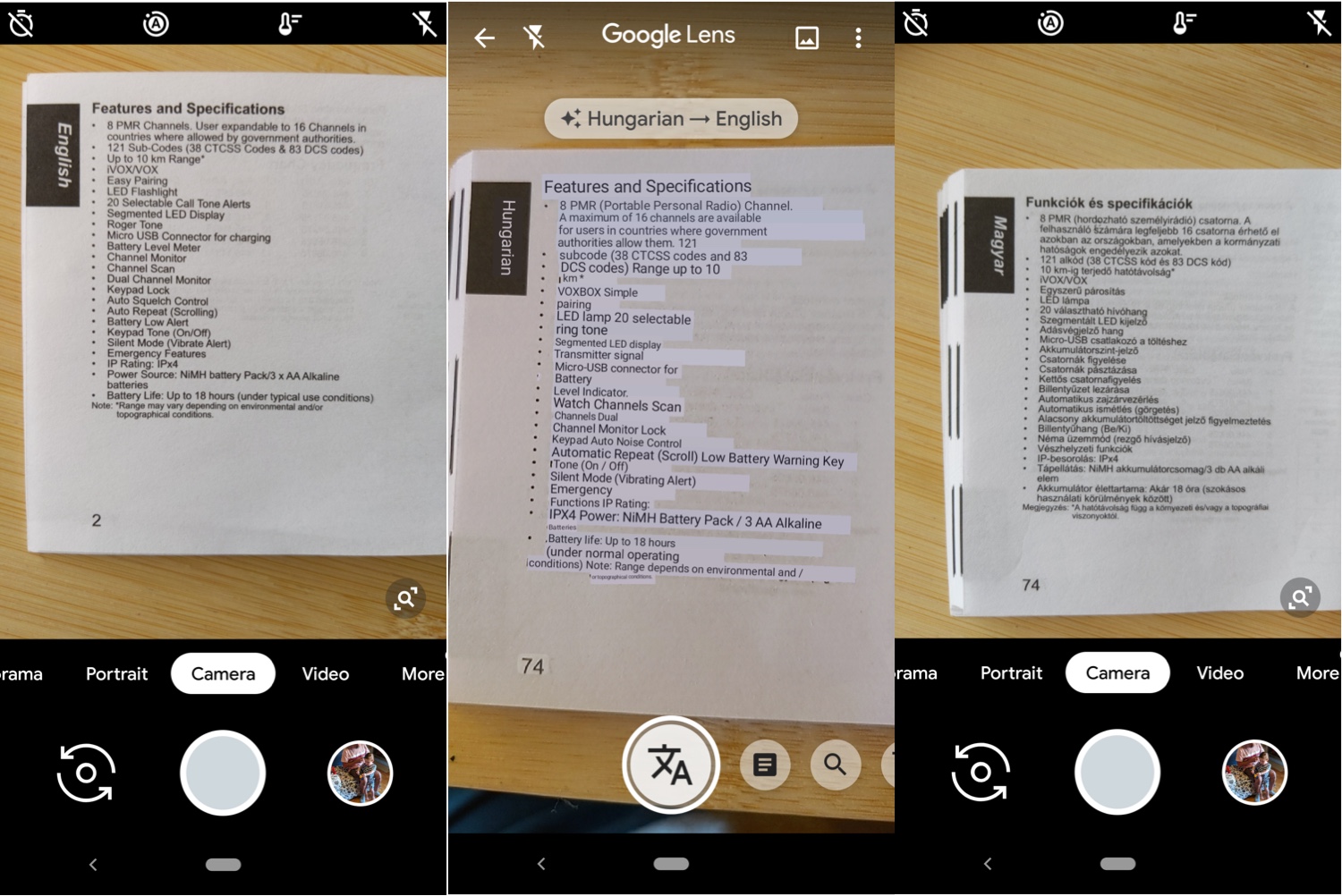
Picture 5.1.3: On the left is a page in English from a Motorola instruction manual. On the right is the same set of information in Hungarian. In the center is the Instant Camera translation from Hungarian to English, as produced with Google Lens software on a Google Pixel 3 camera.
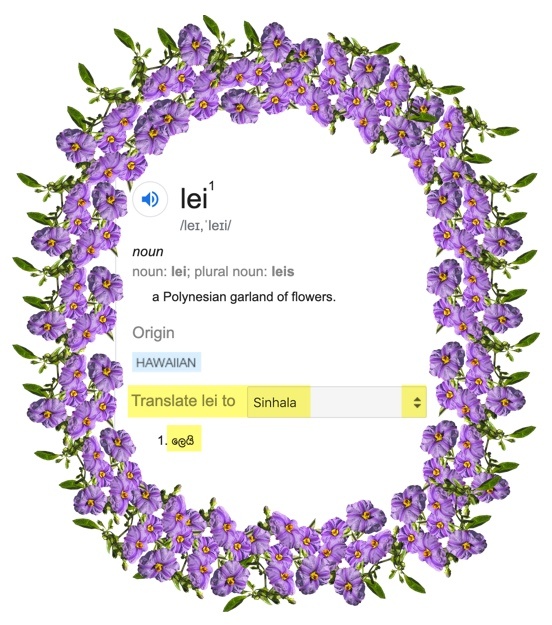
Picture 5.2: The lei lie. GT asserts that “ලෙයි”, which spells the sound “lei”, is a real word with the same meaning. This exercise can be repeated in every Google language as an example of MUSA, the Make Up Stuff Algorithm. (In the rare case that “lei” has been adopted into a language you know, such as Dutch, you can find the same phenomenon with any of bajillions of terms that do not appear in the parallel training vocabulary, such as “bro hug” or “bajillion“).
MUSA: The Make Up Stuff Algorithm24
English is by far the language with the most investment and the most NLP resources, even serving as the primary stomping grounds for computational linguistic research in Europe, Asia, and Africa because that’s where the datasets are and that’s where the jobs are . We all know that Google gets English wrong much of the time, pointing us to off-target search results or silly Android text predictions, but we let ourselves be snookered into believing that they have brilliant algorithms within and among scores of other languages – what we can call BEDS, Better-than-English Derangement Syndrome (see Picture 5.1). Google does have an algorithm that it invokes regularly to generate the aura of brilliance. I call it MUSA, the Make Up Stuff Algorithm. If GT does not find an equivalent for “porn” in its Latin vocabulary, it invokes the MUSA imperative to produce, caveat emptor, “Christmas cruises”.
One genre of MUSA is the “lei lie”. The term “lei” has entered English as a result of the historical relationship between Hawaii and the United States of which it now forms a part, but it is not a universal term in all languages. Nevertheless, GT will provide a “translation” for “lei” in every language in its system, for example “ලෙයි” in Sinhala (Picture 5.2) and “லெய்” in Tamil, the languages of more than 21 million people in Sri Lanka. Both of those are merely phonetic transliterations of the sounds in “l-e-i” in their respective alphabets, not actual words with actual meaning. Yet, not only does GT assert that the glyphs represent real terms in those languages, but offers “லெய்” as a translation of “ලෙයි”. Sri Lankans enjoy flower garlands – one could visit Lassana Flora in Colombo and purchase what transliterates as “mal mala” in Sinhala or “maalai” in Tamil, but GT’s confidence in declaring that such things are called something like “lei” in any language you ask is a confidence scam, a.ka. fake data, a.k.a. a lie.
You could spend all day finding examples of lei lies and other prevarications for all 108 GT languages. Here is a little game derived from an amusing tweet. Using Spanish as your source language, pick an English word that is not a Spanish cognate, and plug it into the blank: “No _______o.” For example, No understando, or No mouseo. Now, choose any target language you can make some sense of. GT will apply some rule from its Spanish repertoire, and throughput English to give you plausible-sounding results in your target language. “No mouseo” comes out in Galician with the rough meaning, “I don’t do mouse”, and in Esperanto, Frisian, German, Swahili, and many other languages as “I am not a mouse”. In French it comes back as “I do not smile” because “souris” is the French word for “mouse”, GT invents “je souris” as the first person conjugation of “to mouse”, and “je souris” just happens to already be the real first person conjugation of “to smile”. Feel welcome to share similar MUSA tests you discover for other languages in the Comments section below.
An MT research scientist at one major company producing public translations, who asks not to be named, suggests that MUSA may be driven in part by consumer demand. They tell TYB, “A translation provider is expected to translate. The user insists on getting something, even if it’s garbage.” This could explain the imperative designers of MT engines feel to provide random words instead of a frank [unknown] label that some portion of a text is outside their confidence zone. Potemkin did not build fake village facades because he liked building fake villages, but because Catherine the Great liked seeing pretty villages as she cruised along the river. Whether consumers really want MUSA may or may not be true, but if service providers think it is true, that helps explain why they algorithmically cover up missing data with fake data.
With no counter-narrative explaining what the hype gets wrong – that GT in fact uses MUSA to invent a French verb, “snooker” as a translation for “snookered”, and then conjugates it by proper rules as “a été snooké“! – most people will assume that the hype is the truth. The research presented herein is the first major attempt to investigate what is true in the claims you hear about GT, what should be taken with salt, and what is pure snake oil.
0.0006% of the Way to Universal Translation25
The tale that GT provides universal translation breaks down even further when you look outside the 108 languages it claims to service. Stated categorically, GT completely fails in 99.9994% of possible translation scenarios. With no service and no known plans26 for 7009 of the world’s 7117 languages that have been granted ISO 639-3 codes (see McCulloch for insight into why most languages remain unserved), “universal” is nowhere on the agenda – neither between any of those languages and English, nor in almost the entire 25,000,000 potential pairs.
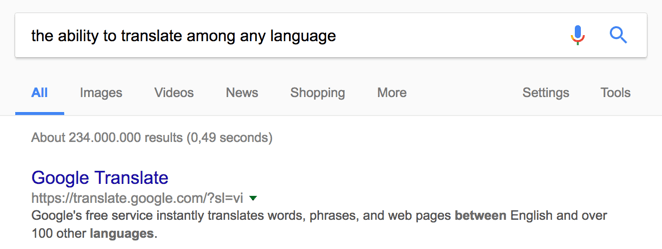
Picture 6: Google’s top result for “the ability to translate among any language” is categorically false, but feeds its own hype.
Granted, first, the languages claimed in GT are spoken to at least some degree by a good half the world’s population,27 and second, many languages on the long tail will never have occasion to come in contact, but leaving the languages of smaller or less powerful groups out of the notion of the quest for “universal” contributes to their further marginalization, and, it could be argued, often works toward their extinction. It is of course not Google’s responsibility to attempt such a feat, but it falls under the rubric of their corporate mission statement (see Picture 7), and they have gotten significant mileage for the publicity surrounding their modest corporate contribution to the Endangered Languages Project28 e.g. . It is the corporation’s responsibility to debunk the impression they let float that they are organizing comprehensive linguistic information and making it universally accessible and useful within MT for most of the world’s languages.
Original Dutch text | Professional human translation | Google Translate translation | Tarzan | BLEU score |
1. Chef-staf Witte Huis John Kelly stapt op | White House Chief of Staff John Kelly steps down | Chief of Staff White House John Kelly gets up | ❌ | 23.74 |
2. AMC kocht honderden hoofden van omstreden Amerikaans bedrijf | AMC bought hundreds of human heads from controversial American company | AMC bought hundreds of heads of controversial American company | ☢ | 48.96 |
3. Russische mensenrechtenactiviste van het eerste uur overleden | Life-long Russian human rights activist has died | Russian human rights activist died from the very beginning | ☢ | 29.07 |
4. Week in beeld: nog meer gele hesjes, goedheiligman en George Bush | Week in pictures: even more yellow vests, Saint Nicholas, and George Bush | Week in the picture: even more yellow vests, good saintman and George Bush | ☢ | 49.36 |
5. Oorzaak Italiaans nachtclubdrama mogelijk ingestorte balustrade | Collapsed railing possible cause of Italian nightclub drama | Cause Italian nightclub drama collapsed balustrade | ✅ | 19.74 |
Table 1: Comparison of human and machine translations of 5 headlines from the leading Dutch television news program NOS Journaal, as they appeared online on December 8, 2018. Scores calculated by the Interactive BLEU score evaluator from Tilde Custom Machine Translation. ❌ indicates a translation from which the original sense cannot be extracted. ☢ indicates a a translation where a speaker of the target language will understand some of the original sense. ✅ indicates a translation where a speaker of the target language can fully understand the original sense. |
Ideology and Computer Science29
MT is in some ways made harder by the ideology of computer science (CS). Computer science sees translation as a computational problem. When the algorithms are perfected, the thinking goes, we will achieve the goal. We have done this before: we built ships to cross the oceans, airplanes to break the sound barrier, rockets to escape gravity; we harnessed electricity, invented the radio, created the Internet. With enough spirit and enough effort, we can achieve any goal we can identify.
For language, the evident goal is the ability to input any language and have human-quality output in any other. We have already witnessed impressive feats along that path, so a few more tweaks and we will most certainly arrive. We will, so it is thought, reach the day when any linguistic expression such as the Dutch sentences in Table 1 can be converted by machine to the caliber of the human translations in the second column. In CS ideology, the actual outputs that machines currently produce, like the GT translations in Table 1, are merely the messy residue of the construction phase, soon to be remembered along with CRT monitors and dial-up modems.
Success in MT is measured by the “BLEU” score (discussed in more depth in the qualitative analysis) – rating whether the machine got a lot of the same words as a human and whether they were arranged in a similar order – despite, as Sentence 2 in Table 1 shows, the entire semantic value of a translation being rendered unintelligible by an error as small as “of” instead of “from”, and despite nuances such as the difference between calling a country “Macedonia” or “North Macedonia” (see Picture 16) being the subject of a costly 28 year international dispute. Based on BLEU score, the big problem with Sentence 1 in Table 1 is GT’s semantically irrelevant word order of “Chief of Staff White House”, which would raise the BLEU score by 42.31 points to 66.05 if fixed. Changing “gets up” to “resigns” would only nudge BLEU up by 0.39 points, to 24.13, although that is the semantic crux of the sentence; shrinking the translation to two words, “Kelly resigns”, would carry all the information needed for a clear translation, but would sink the BLEU score to 4.38. That is, the difference between the failure or the success of a translation for a consumer is the difference between “gets up” and “resigns”, whereas to a computer scientist it is the difference between 4.38 and 66.05. This is not to disparage interesting work that might one day contribute to superior MT, but when results with BLEU between 9% and 17% are reported as “acceptable” , we must be clear that this refers to acceptability in pushing the envelope from an experimental standpoint, not from the standpoint of linguistic viability.
People have natural intelligence that makes any human with normal brain functioning capable of learning, say, Bengali (the world’s seventh most-spoken language). No human who has not learned Bengali is capable of translating it, however. Similarly, in principle, any computer could perform in Bengali the full gamut of things we ask computers to do in English. In practice, though, without substantial Bengali data, even the most sophisticated supercomputer will never be able to make the language operational. Allusions to cognitive neuroscience – AI (Myth 1), deep learning, neural networks (Myth 2) – along with false claims about the success of “zero-shot translation” (Myth 3), produce the illusion that effective translation for any language is a few algorithmic tweaks away. The fact is that no adequate digitized data exists for more than a smattering of lucrative languages so MT is impossible in most circumstances. You cannot get blood from a stone, no matter how brilliant your code base. Though GT will undoubtedly eventually add some more languages to its roster (indigenous American languages being conspicuous in their absence), a new approach that builds upon natural intelligence is needed if the supermajority of languages are ever to participate in the information age.
In-depth research into the potentials for universal translation, of which the present study is a part, points to a conclusion that flies in the face of the prevailing computer science ideology. I find that computers are indeed a powerful tool that can perform much of the grunt work of translation, and do so for many more languages than FAAMG has any current plans to support. “Computer Assisted Translation” (CAT), wherein the machine proposes a sheaf of text such as Sentence 4 in Table 1, and the person separates the wheat from the chaff by post-editing, is already used seriously by professional translators. The disruptive approach I outline here , though, will strike many as heretical, or at the least fantastical. I propose that meaning can be ascribed in the source language – “goedheiligman” can be known to be the idea of Saint Nicolas – before a text ever reaches the eyes of a translator. This can be achieved for even the smallest languages, by collecting data from human participants through systems under development, and using text analysis tools already under development, at costs remarkably lower than what has been invested over decades in languages like English and Dutch. Reaching that level – where real translation among hundreds of languages occurs by using MT as a chisel in the sculptor’s hands, not the sculptor itself – can be realized, but is only possible with a paradigm shift that drops the pretense that true translation can ever be fully automated.
MT is perhaps better seen in the first order as a social science problem – how to collect the language data that machines can process in the first place, and how to present the collected data in a way that people can refine for final translations. More than two hundred million people store a full Bengali dataset and processing algorithms in their heads. For Bengali and thousands of other languages, computers can be used as the tool to collect the data from people, in a format that establishes parallel concepts across the board. Once people provide data, computers handily store and perform the mechanical tasks of regurgitating it. Machines can further be set to work analyzing the data to find patterns that might not be apparent to humans. This is where AI comes in: to flag cross-linguistic correspondences requires a person to be well-versed in two languages, but only requires a computer to have comparable data. It then remains for the computer to put its propositions before human eyes, and for the humans to render final judgement.
GT has achieved demonstrable success in reaching somewhere on the spectrum between silence and true translations for the languages it covers – a worthwhile goal, as long as it is understood that the output is a fictional set of sometimes-informed guesses. In theory, computation could attain competent Tarzan-level translations between any language pair that is supplied with a large stock of comparable data. The argument in this study is not that universal translation cannot be accomplished, but that the empirical results prove that GT has not accomplished it, and analysis of their methods prove that it cannot be accomplished with the methods that FAAMG are currently pursuing.
![]() |
![]() |
![]() |
GT output is built on three pillars. The first pillar is known facts about language, including the internal structures of the source language and confirmed correspondences to the target language – hard wiring, as depicted in Picture 8. The second pillar can be represented by the rolling of multisided dice, as depicted in Picture 9, where the machine gambles from numerous possibilities based on some sort of prediction from nebulous data. The third pillar, depicted in Picture 10, is pure air, packed in the output box in order to fill space. Crucially, GT employs all three of these techniques in all of their languages, often within the space of a single sentence. The research presented in the empirical evaluation gives an indication of how the weight of each language is balanced atop the pillars of knowledge, prayer, and pixie dust.
References
Items cited on this page are listed below. You can also view a complete list of references cited in Teach You Backwards, compiled using Zotero, on the Bibliography, Acronyms, and Technical Terms page.
To notify TYB of a spelling, grammatical, or factual error, or something else that needs fixing, please select the problematic text and press Ctrl+Enter.
Footnotes
- As in the documentary Icarus, where the initial limited exploration of doping among competitive bikers unveiled a fraud that permeated Russian sports.
- “Citizen science” typically refers to research collaborations in which volunteers partner with scientists to answer real-world questions. Defining Citizen Science: http://www.birds.cornell.edu/citscitoolkit/about/definition
- Pedants please stand down. Data as singular is valid contemporary English, as confirmed by the National Science Foundation program officer at the Office of Science and Technology Policy in charge of including Kamusi within the White House Big Data Initiative in 2013.
- link here: http://kamu.si/tyb-overview
- Aiken and Balan (2011) conducted an analysis of 7 test sentences in the 2010 version, when GT had 51 languages and was using an earlier statistical translation model, using comparative BLEU scores rather than human evaluations as their metric.
- To wit, a conversation with a Romanian computer scientist who was talking about the high quality of products from Google. He enthusiastically mentioned that one could use GT to translate from Italian to French. When asked whether he spoke Italian, he said no. Nor had he tried using GT for this language pair. Nor would he use GT to translate from Romanian to English or French, because his own capacity in those languages surpassed the results he would get from GT. However, the dropdown menus on GT give him the option to choose Italian as the source language and French as the target. His overall confidence in Google thus led him to assume that GT translations between the selected languages would be accurate. This anecdotal data point is typical of many reactions the I have noted, and which have spurred the current study to answer the question empirically of how valid this assumption actually is.
- link here: http://kamu.si/tyb-tropes
- link here: http://kamu.si/trope-1-elite-languages
- link here: http://kamu.si/trope-2-English
- Pro tip: if you need urgent help with English while you are traveling, try finding a pharmacist, because that is one profession where exams are often in English. At least, this is true for countries that spent time under the control of the British Empire, such as Egypt.
- link here: http://kamu.si/trope-3-trust
- link here: http://kamu.si/trope-4-disbelief
- link here: http://kamu.si/trope-5-perfect
- How Accurate is Google Translate in 2019?
- The Surveillance Is Coming From Inside the (Smart) House (minute 23)
- link here: http://kamu.si/trope-6-learns
- link here: http://kamu.si/trope-7-gaps
- link here: http://kamu.si/trope-8-confirmation
- link here: http://kamu.si/trope-9-gaslighting
- link here: http://kamu.si/trope-10-hype
- The only service this study evaluates at an empirical level is GT. However, much of the conceptual discussion also pertains to other competitors within the MT space, including Facebook, Bing, and DeepL.
- Facebook’s translation to English of a post on the wall of a Chinese speaker:
到玩具店買姪子的生日禮物,結果被這位先森盧到一盒樂高😂
身為對系列電影無感、連哈利波特看不到十分鐘就進入休眠狀態的無童年女子,我腦裡第一浮現的想法是,為什麼要花好幾天菜錢買一個組了不知道擺哪裡的玩具?然而,結帳時當我看到身後小孩那股無比炙熱的目光、還有手裡抱著這盒、雙眼炯炯有神嘴角還略帶淺笑的嘎嘎,我好像突然理解了什麼。Bought my nephew’s birthday gift at the toy shop, and the result was a box of Lego in the first place. 😂
In a series of films that don’t feel like a series of movies, even harry potter can’t see a woman who is in hibernation for ten minutes. The first idea in my brain is why it took me a few days to buy a group to buy a group. I don’t know. Where are the toys? However, when I saw the very hot eyes of the kid behind me, I also held this box in my hand, the eyes of the eyes, the eyes of my eyes, and the quack quack, I seemed to understand something suddenly. - link here: http://kamu.si/instant-camera
- link here: http://kamu.si/tyb-musa
- link here: http://kamu.si/tyb-not-universal
- It will be no surprise if one day GT announces the inclusion of a handful or a few dozen more languages. My crystal ball predicts that they are especially working behind the scenes to introduce a few indigenous languages of the Americas.
- Most estimates of the number of speakers of a language are wild approximations because (a) language information is rarely collected in census data, (b) many people speak multiple languages but are only included in estimates for one, and (c) migration means that many speakers of a language will be missed in estimates based on geography. An exception might be an isolated language like Icelandic, where almost all native inhabitants speak the language, and the number of non-Icelandic-speaking immigrants could be guessed to be somewhat equal to the number of Icelanders living abroad.
- The Endangered Languages Project (http://www.endangeredlanguages.com) is a wonderful and important undertaking. It is not, however, designed to collect linguistic data for inclusion in NLP technologies such as MT.
- link here: http://kamu.si/tyb-ideology